Skand
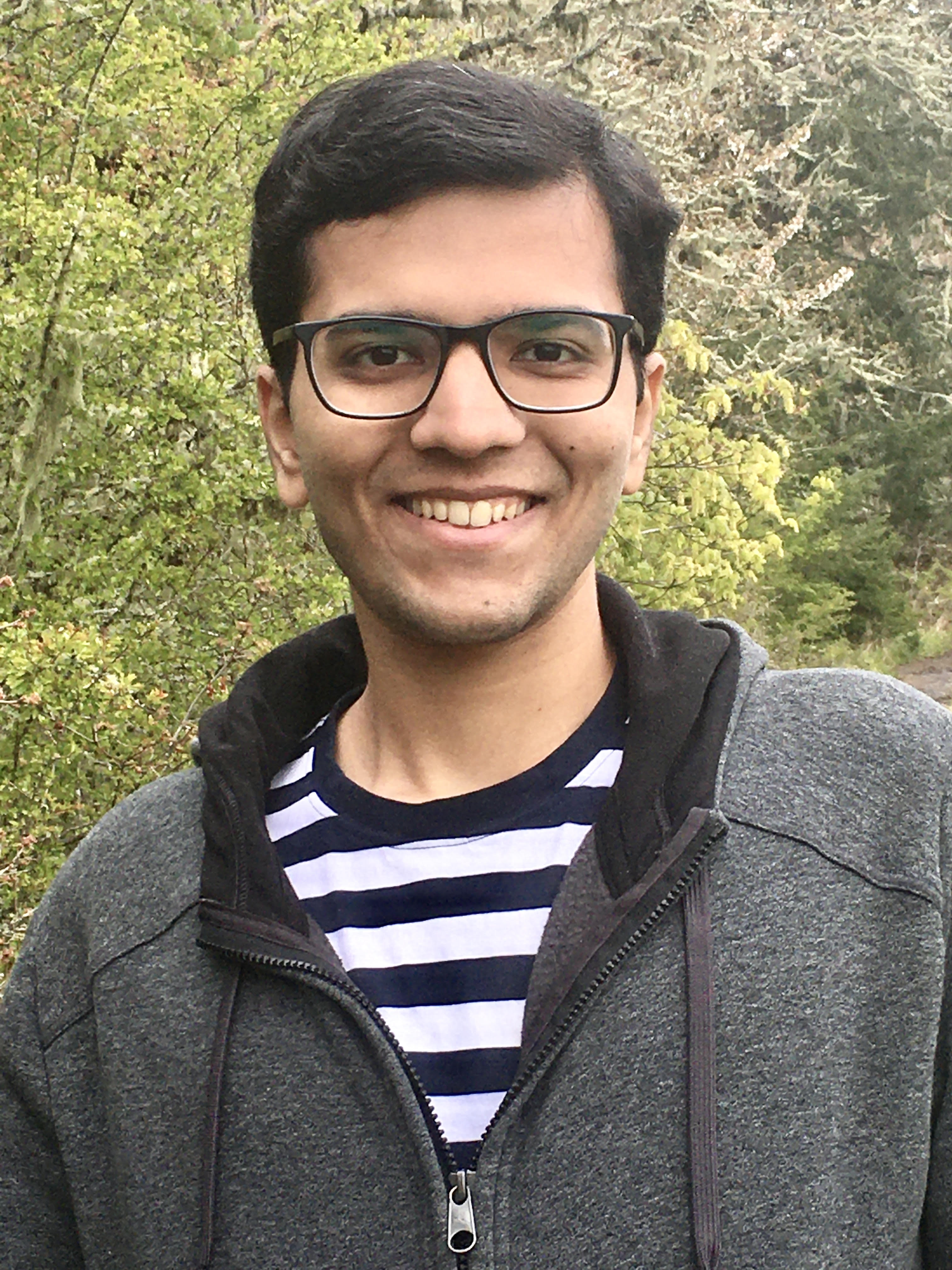
I am a third year Ph.D. student at Oregon State University advised by Fuxin Li and Stefan Lee. My primary research interests lie at the intersection of robot learning and model-based reinforcement learning.
Previously, I worked with Sungjin Ahn on object-centric world models and reinforcement learning. Earlier, I was a Research Assistant at the Indian Institute of Science working with Venkatesh Babu. I graduated with Bachelors of Technology (B.Tech) degree in Computer Science and Engineering from Indian Institute of Technology, Ropar, India.
I was advised by Narayanan Krishnan for my Bachelor’s Thesis. During my undergrad, I also worked with Deepti Bathula and Abhinav Dhall. I previously interned at DeepChem (GSoC) and Video Analytics Lab, IISc Bangalore.